Completed: Improved Energy Efficiency of Float Glass Production
In collaboration with Pilkington NSG
Of all the ‘enablers’ of transformation, digitisation and control offers the greatest potential to impact across multiple FI sectors and its benefits of reduced plant downtime and energy and raw material savings are clear. Implementation however, deviates significantly from the classic skill sets associated with the foundation industries, requiring knowledge of advanced computing, sensing, modelling and data handling.
In the built environment, glass is pivotal in modern construction with ten million tonnes of float glass produced in Europe every year, with the UK currently having a 6% share of European glass production. Pilkington United Kingdom Limited, part of NSG Group, is a leading UK glass manufacturer and supplier with around 3,000 staff across the UK and producing a wide range of glass solutions (glass for architectural applications, solar panels, automotive equipment, touchscreens etc.) Being an energy-intensive manufacturing process and noting NSG Group’s aim to achieve a 30% reduction in CO2 emissions by 2030, this project explored the development of a data-based model which can, ultimately, be used to increase the efficiency of the float glass production.
This study involved the development and deployment of a machine learning model which, having been trained on data gathered from 100s of sensors at the UK5 float glass plant in St. Helens, was used to predict variations in product quality up to 72 hours into the future. The overall model was formulated using a Gaussian ‘product of experts’ approach, whereby separate models are each used to cover different regions of the input space before predictions are formulated using a weighted product of all models. The figure below is an example fault density prediction model which is used to improve efficiency of the float glass process.
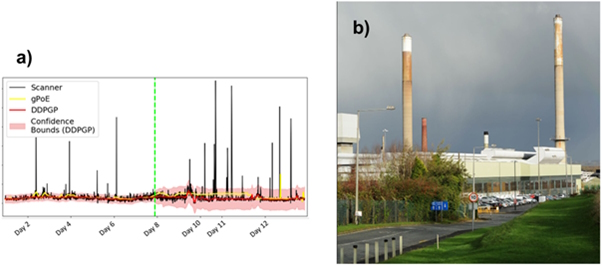
The model is able to function in an online setting (allowing it to be updated using the most recent data from the UK5 plant). The model can be used to form different decision support tools (e.g. the optimiser, sensitivity analysis) to help increase the efficiency of float glass production. The approach is scalable to large datasets and, to the best of our knowledge, generic in that it can be extended to other float glass furnaces. NSG has directly funded another 6 months of research which has already been transferred into the Manufacturing Execution System used at the UK5 plant.
Publications
Predicting product quality in continuous manufacturing processes using a scalable robust Gaussian Process approach, https://doi.org/10.1016/j.engappai.2023.107233
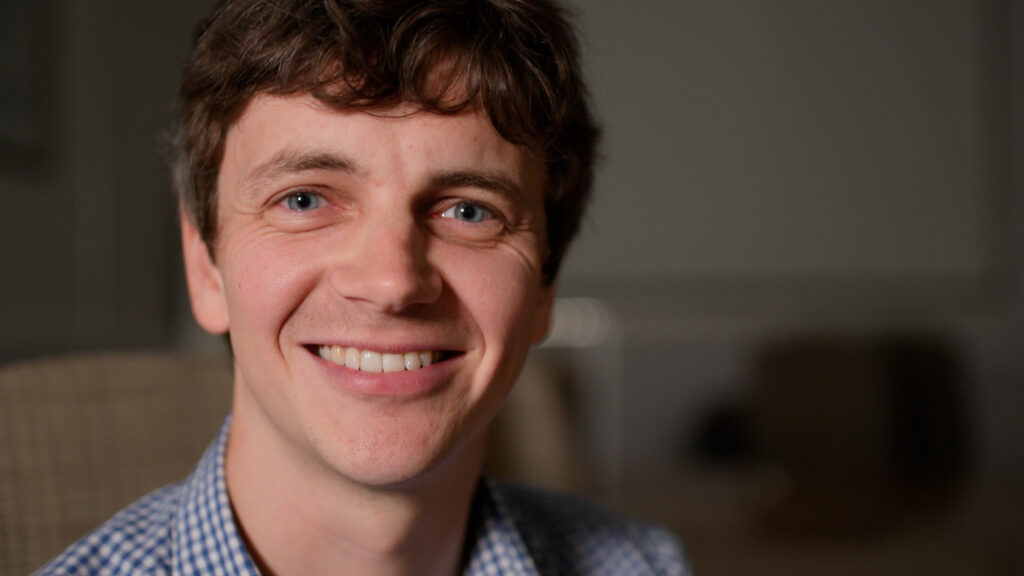
Dr Peter Green
University of Liverpool
Email: P.L.Green@liverpool.ac.uk
Dr Green obtained a PhD in mechanical engineering at the University of Sheffield in 2012, before becoming a lecturer at the University of Liverpool in 2015. He is currently a senior lecturer, also at the University of Liverpool. Dr Green’s background is in structural dynamics, and the development of models whose parametric uncertainty can be analysed using Bayesian approaches. This has
since been expanded into the general development of machine learning (a.k.a. AI
) models for engineering applications. His fundamental research concerns the development of data-based models that facilitate uncertainty quantification and are scalable to large datasets.
Published: November 3rd, 2021
Posted in
projects