Completed: Physics-informed digital twins for industrial heating processes (twin4heat)
In collaboration with VESUVIUS and SWERIM
Twin4heat’s ambition was to accelerate the UK’s Net-Zero trajectory through next generation industrial heating processes using artificial intelligence to break through the predictive capability bottleneck of most surrogate models due to time consuming data acquisition in thermal analysis, design and optimisation. It aimed to tackle the challenge of automatic, fast, and frequent temperature prediction and setpoint estimation tasks (the most wide-spread tasks in the intelligent industrial heating process) by embedding physics knowledge of heating processes into Artificial Neural Networks.
The project studied the problem of predicting temperatures within a digital twin model of an industrial heating system, such as the reheating furnaces, via modelling the underlying physical phenomenon of radiative heat transfer. The first contribution is to cast the prediction problem as a regression task to employ Machine Learning (ML) based methods. Then, physics-based regularization terms derived from the classical Hottel’s zone method are proposed for equipping a neural network with better generalizability. Evaluating the proposed approach against a range of ML baselines using simulated data from a real-world furnace showcased the exceptional capabilities of neural networks to leverage the benefits of physical knowledge. Twin4Heat’s ground-breaking methodology holds tremendous potential for transforming industrial heating processes, enabling substantial advancements in energy efficiency, emissions reduction, sustainability, and profitability within the foundation industries.
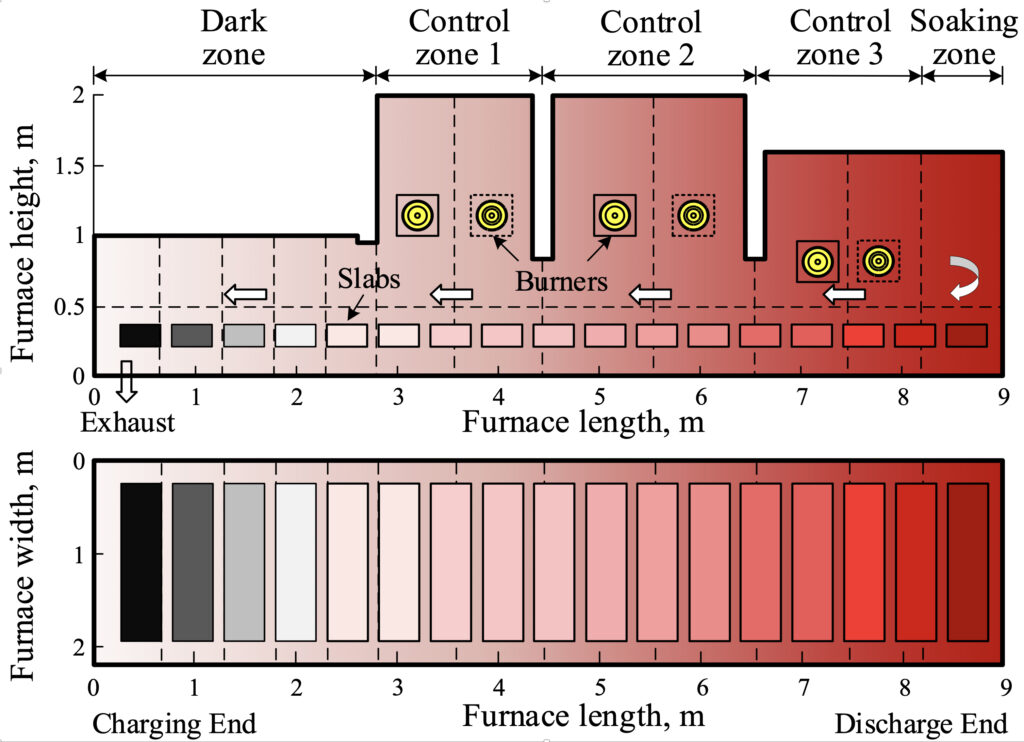
Publications
- Applications of Agent-Based Methods in Multi-Energy Systems—A Systematic Literature Review, https://doi.org/10.3390/en16052456
- Application of Zone Method based Physics-Informed Neural Networks in Reheating Furnaces, https://doi.org/10.48550/arXiv.2308.16089
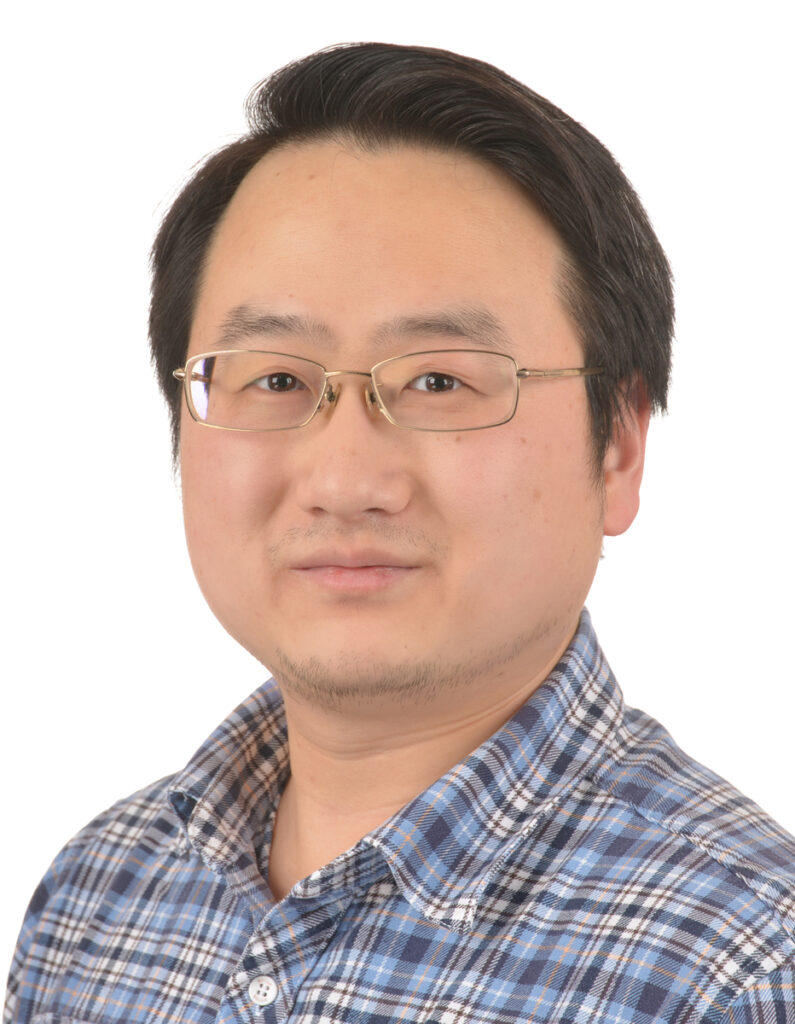
Dr. Yukun Hu
University College London
Published: September 23rd, 2022
Posted in
projects